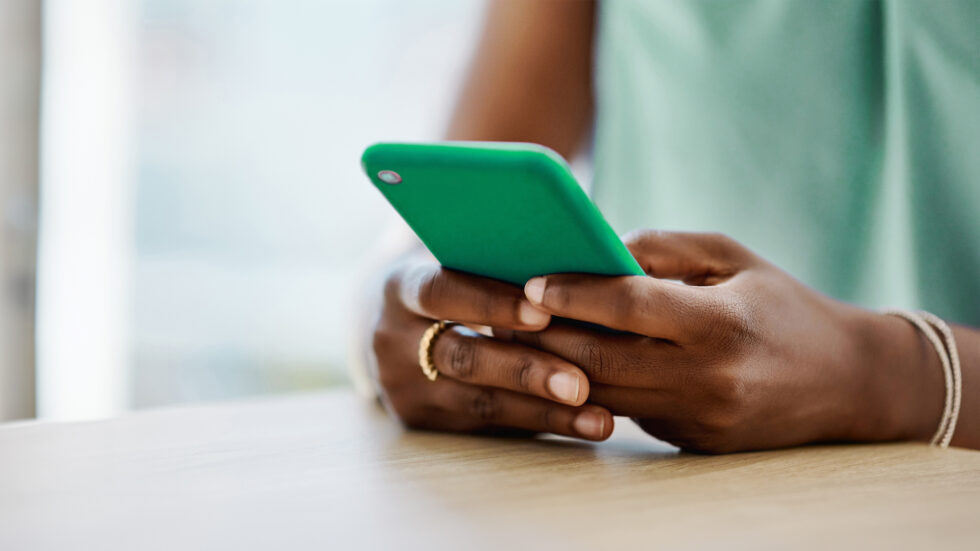
Women tend to have better loan repayment rates than men. There is an implication that women are subject to a stricter loan application evaluation. Loan officers could be gender-biased, there may not be sufficient data for women, or data sources may not fully account for gender differences, creating barriers to loan approval. In such cases, lending models could be adjusted to improve risk assessment and increase financing to women.
A guide by CGAP, a think tank focused on the betterment of the lives of the poor though financial services, shows that a disaggregated gender analysis of a loan portfolio can unveil potential gender-intentional strategies to grow both the total loan book and the share of women borrowers without increasing the portfolio’s credit risk.
A simple loan portfolio analysis by borrower’s gender and loan outcome can show the relative share of loans to women and their loan repayment. However, a credit scorecard, or other similar tools that differentiate clients, such as rating or segmentation models, will be needed to develop and implement gender-intentional credit risk management strategies.
Credit score information helps lenders to make a more precise choice in their approval process. A gender-blind or gender-intentional loan approval strategy could be used to lower “bad” rate on loans, with no change to the credit scoring model itself.
When past “bad” rate on loans to women is lower than on loans to men, gender-intentional strategies accurately align scorecards with risk appetite and ensure the maximum number of women borrowers are reached for any given risk target by setting separate scoring decision thresholds by gender. Although these methods require credit scorecard development capabilities, they are no more complex than developing a gender-blind scorecard.
Under a gender-intentional strategy, gender data can explicitly be considered when building scorecards either by using gender as a scorecard characteristic or by building separate scorecards for women and men. Building separate scorecards for men and women could potentially result in better outcomes for each group. However, it requires a rich dataset.
The publication highlights exemplary use cases. For instance, AB Bank Zambia (ABZ) increased the share of microloans to women from 57% to 60% by including gender as a scorecard characteristic. More interestingly, the bank lowered its women’s “bad” rate from 5% (versus 7% for men) to 3.5% (versus the same 3.5% for men). This shows that the additional points women borrowers received at the scoring stage accurately reflected their better past repayment, correcting the gender-blind score and setting the risk bar equally for men and women.
Besides increasing lending to women, gender-intentional strategies can help lenders to better understand how men and women borrowers differ and examine ways to improve lending outcomes for women. For instance, they could be used for risk-based pricing to lower interest rates for women borrowers, making loan payments more affordable. This reveals that there are more accurate ways to increase lending to underserved groups while creating business value for providers.